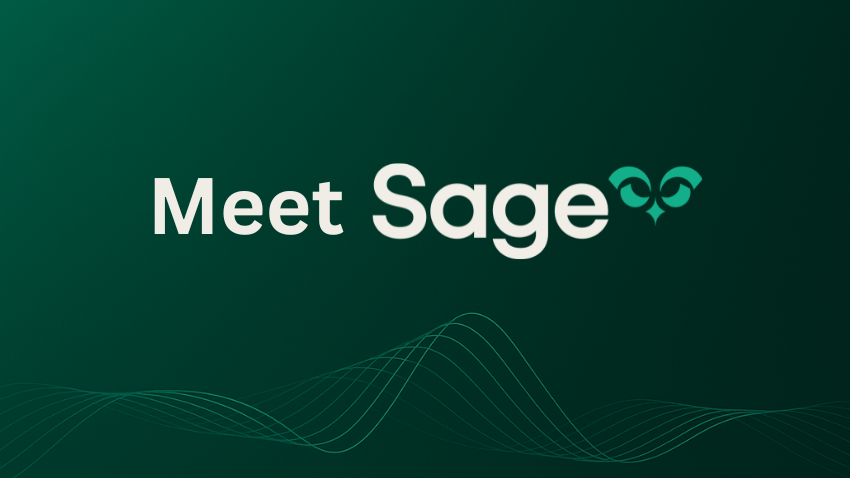
Meet Sage, the AI Care Manager
Today, we announced something we’ve been working toward for years: Sage, our emotionally intelligent AI Care Manager, along with $45 million in funding from CVS Health Ventures, Salesforce, Khosla Ventures, and our existing investors.
All Types
- Blog
- News
- Research
- White Papers
- All Types
All Topics
- Blog
- In the News
- Press Release
- Research Paper
- Video
- All Topics